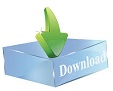
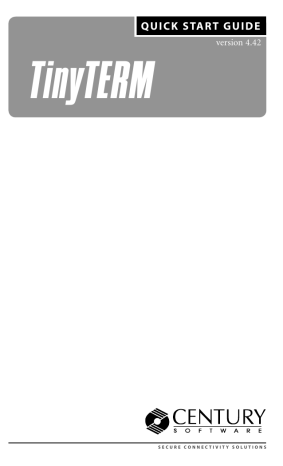
TinyTERM’s industrial browser includes comprehensive scanning capabilities for scanning bar codes into input fields, redirecting QR scan codes to web pages and full compatibility with Safari. TinyTERM Enterprise provides enterprise deployment capabilities including configuration push, user interface lockdown and extensive configuration import/export management. The included secure SSH, SSL, and telnet IBM/VT/ANSI/Wyse/ADDS terminal emulation provides macros, printing and automation capabilities with scanner support. Crucially, analyses of the training dynamics of these methods reveal that they can meta-learn the correct subspace onto which the data should be projected.TinyTERM Enterprise for iOS combines secure terminal emulation with highly configurable keyboards to allow creation and deployment of purpose-based and scanning devices requiring access to legacy hosts or HTML 5/Javascript application in modern mobile environments. The `Fr\'(1)$, demonstrating a separation from convex meta-learning. Prefers flat minima in the objective landscape.įor the evaluation of the performance of GANs at image generation, we introduce Prove that it follows the dynamics of a heavy ball with friction and thus The convergence carries over to the popular Adam optimization, for which we Prove that the TTUR converges under mild assumptions to a stationary local Nash equilibrium. Using the theory of stochastic approximation, we
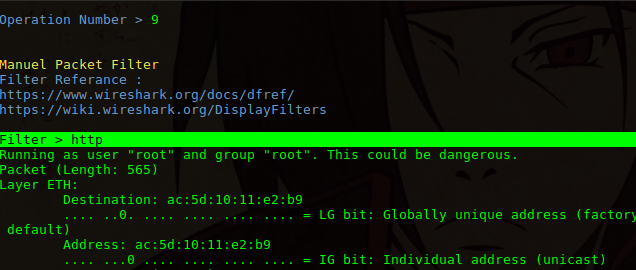
TTUR has an individual learning rate for both the discriminatorĪnd the generator. Gradient descent on arbitrary GAN loss functions.
#Tinyterm capture file update
We propose a two time-scale update rule (TTUR) for training GANs with stochastic However, theĬonvergence of GAN training has still not been proved. Generative Adversarial Networks (GANs) excel at creating realistic images withĬomplex models for which maximum likelihood is infeasible. Finally, our method can generate high-fidelity images using only 100 images without pre-training, while being on par with existing transfer learning algorithms. Furthermore, with only 20% training data, we can match the top performance on CIFAR-10 and CIFAR-100. With DiffAugment, we achieve a state-of-the-art FID of 6.80 with an IS of 100.8 on ImageNet 128x128 and 2-4x reductions of FID given 1,000 images on FFHQ and LSUN.

Experiments demonstrate consistent gains of our method over a variety of GAN architectures and loss functions for both unconditional and class-conditional generation. Previous attempts to directly augment the training data manipulate the distribution of real images, yielding little benefit DiffAugment enables us to adopt the differentiable augmentation for the generated samples, effectively stabilizes training, and leads to better convergence. To combat it, we propose Differentiable Augmentation (DiffAugment), a simple method that improves the data efficiency of GANs by imposing various types of differentiable augmentations on both real and fake samples. This is mainly because the discriminator is memorizing the exact training set. The performance of generative adversarial networks (GANs) heavily deteriorates given a limited amount of training data. In addition to a new approach for predicting generalization, the counter-intuitive phenomena presented in our work may also call for a better understanding of GANs' strengths and limitations. Several additional experiments are presented to explore reasons why GANs do well at this task. Yet the generated samples are good enough to substitute for test data. mode collapse) and are known to not learn the data distribution accurately.
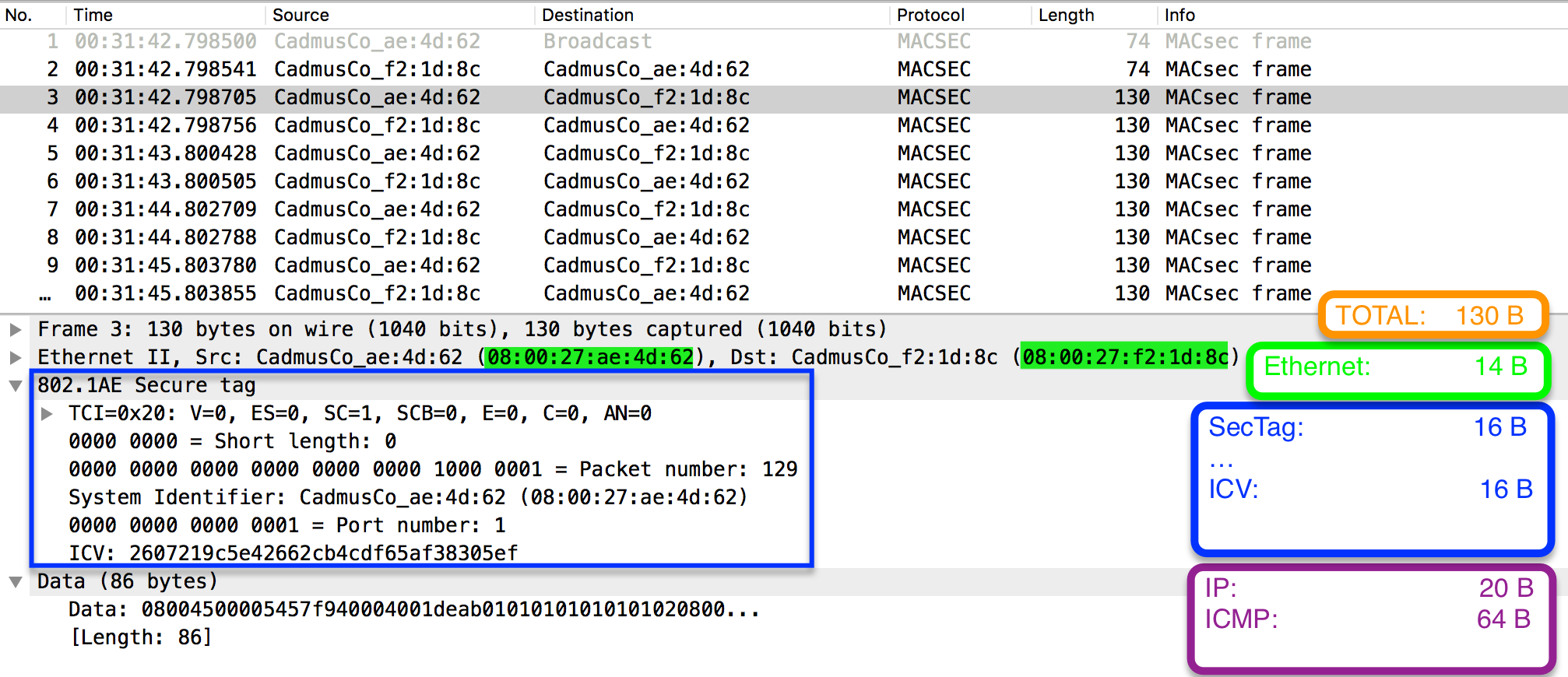
This result is surprising because GANs have well-known limitations (e.g. In fact, using GANs pre-trained on standard datasets, the test error can be predicted without requiring any additional hyper-parameter tuning. The current paper investigates a simple idea: can test error be predicted using 'synthetic data' produced using a Generative Adversarial Network (GAN) that was trained on the same training dataset? Upon investigating several GAN models and architectures, we find that this turns out to be the case. A recently introduced Predicting Generalization in Deep Learning competition aims to encourage discovery of methods to better predict test error. While generalization bounds can give many insights about architecture design, training algorithms etc., what they do not currently do is yield good predictions for actual test error. Research on generalization bounds for deep networks seeks to give ways to predict test error using just the training dataset and the network parameters.
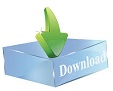